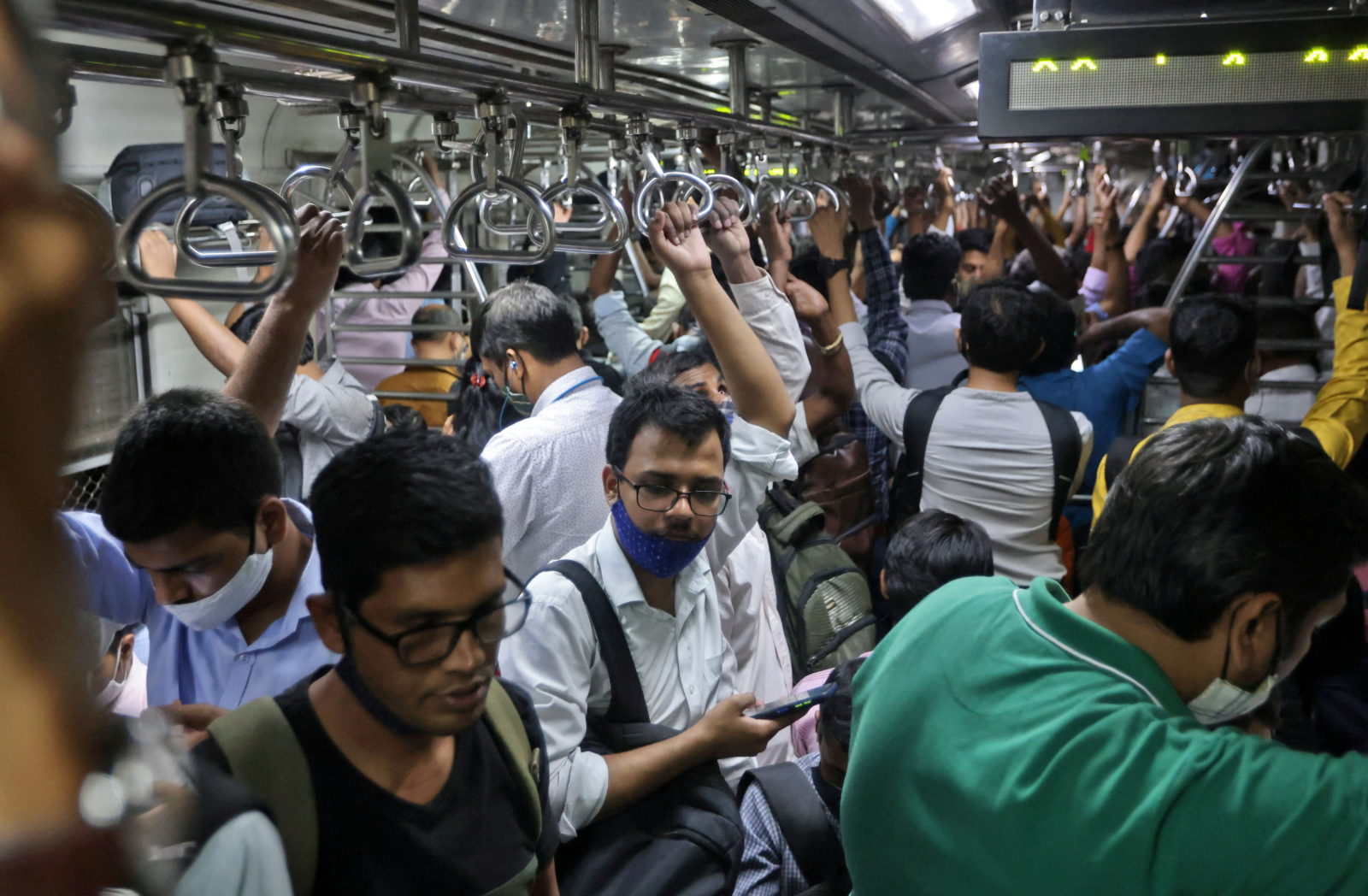
Commuters travel in a packed train in Mumbai, India, February 25, 2022. Photo: Reuters/Francis Mascarenhas
- A team of researchers from IIT Kanpur have predicted a fourth COVID-19 wave in India from June 22 to October 24, probably peaking in August.
- However, the model doesn’t account for characteristics of a new variant, its immune escape potential and vaccination and booster dose coverage, among others.
- The forecast is also for four months in one go. As a result, it is inhospitable to unexpected changes, which are almost certain to happen.
New Delhi: As India continues to ease restrictions against the COVID-19 epidemic and people are relieved in the wake of the omicron variant wave, IIT Kanpur researchers uploaded a study predicting a fourth wave between June and October.
Modelling, as a concept, is not new in science. But it became much more prominent in the last two years, paralleling the COVID-19 pandemic.
Every time modellers released a new prediction, especially in India, it elicited broadly two kinds of response from the people at large: fear and sheer disbelief. Many people have been sceptical about modelling since the pandemic began – more so when models failed to predict India’s big second wave.
After this, models became more polarising. Those who usually got scared tended to believe the predictions completely. The other group tended to reject models’ predictions, pointing, among other instances, to their failure to anticipate the second wave.
Even if neither is entirely right, neither is entirely wrong either.
How much should one really rely on modelling?
The IIT Kanpur study, released on February 24, said India will have its fourth wave between June 22 and October 24, and probably peak in August.
Brian Wahl, who, among other things, uses models to study infectious diseases at Johns Hopkins University’s Department of International Health, told The Wire Science that a model could be reliable if it made predictions a week or so into the future. The recent IIT Kanpur model, which used data available until January 30, 2022, makes predictions for a period more than 15 weeks away.
Gautam I. Menon, a professor of physics and biology at Ashoka University, Sonepat, agreed.
“Whatever situation in the pandemic – whether cases are going up or coming down,” researchers build models based on existing data, Menon explained.
“But you do it [modelling] for a week or so, then you do it again as the data changes. And thus you keep refining,” he said. “You don’t project for two months together.”
The IIT Kanpur’s forecast is for four months in one go. Thus, it is inhospitable to unexpected changes, which are almost certain to happen.
This is also not the first model to have predicted the exact dates of a wave. The chairman of India’s national COVID-19 task force, Vinod K. Paul, said in April 2020 that there will be zero COVID-19 cases in the country by May 16, 2020. He was later proven wrong and, to his credit, apologised.
A prediction that includes exact dates is a prediction that is more certain about the future than is possible, at least vis-à-vis infectious diseases.
In fact, our past experiences have also shown that a new wave follows the rise of a new (dominating) variant. “It has never happened because of waning immunity only,” Menon said.
Both Wahl and Menon also said that the IIT Kanpur model failed to combine mathematics with epidemiology. Specifically, they said the model doesn’t account for important epidemiological factors: characteristics of a new variant, its immune escape potential, vaccination and booster dose coverage, paediatric COVID-19 vaccination and adherence to COVID-appropriate behaviour.
In the study’s preprint paper, the IIT Kanpur team says, “Overall, it should be mentioned that the forecast may be affected by all these reasons in the future.”
In news reports of the study, however, this important caveat was often buried below a few paragraphs of text, effectively sidelining it.
In addition, considering omicron remains the dominant variant, the authors could not have overcome these limitations. Therefore, the timing for which projection was done becomes all the more important.
According to Wahl, it’s safest to have a “scenario-based” forecast – that is, predictions for two or three scenarios at a time, depending on potential uncertainties.
“Say, one scenario could be if the new variant is two-times more transmissible,” he explained. “Another scenario could be if it is five-times more transmissible. You make these assumptions and look at different peaks of different magnitudes at different times.”
Menon also had a problem with the preprint paper comparing the COVID-19 scenarios of Zimbabwe and India to conclude that the former is going through a fourth wave.
“Zimbabwe and India have the maximum visible similarities in the shape of the COVID waves, the COVID cases in Zimbabwe [are] considered as the training data set,” the authors have written.
However, Menon said there are big differences between the two countries’ demographic compositions and their vaccination statuses. He tweeted on March 2:
That’s just one part of it. The median age in Zimbabwe is close to 19. For India it is close to 30. The impact of COVID-19 in every respect – cases, ICU-requirements, deaths – are primarily driven by age.
— Gautam Menon (@MenonBioPhysics) March 2, 2022
And according to Our World in Data, a little more than 25% of Zimbabwe’s eligible population is fully vaccinated, versus more than 70% of that in India.
Even the Union government – which has adopted the prescriptions of dubious models in the past – has taken the current one with some salt.
“Only on a single estimate or projection, one is not taking any decision of significance. We value it as an input. Whether it has a scientific worth and mathematical underpinning will be examined,” Paul, the COVID task force chairman, said on March 3.
The quality of any model depends on the existing data that it builds on. If the data is not good, the model won’t be good either. “For example, in India’s case, it is well-known that during the omicron variant’s outbreak, a large number of cases went unreported because testing went down,” Menon said. “So if that data is fed into a model, the results can’t be fully reliable.”
Models can also go awry if a variant with significantly different epidemiological characteristics turns up.
For all these reasons, the usefulness of models lies somewhere between the two reactions: they are not the gospel truth, and they are not entirely dismissable either. Wahl signed off with a quote by the late British statistician George P. Box: “All models are wrong, but some are useful.”