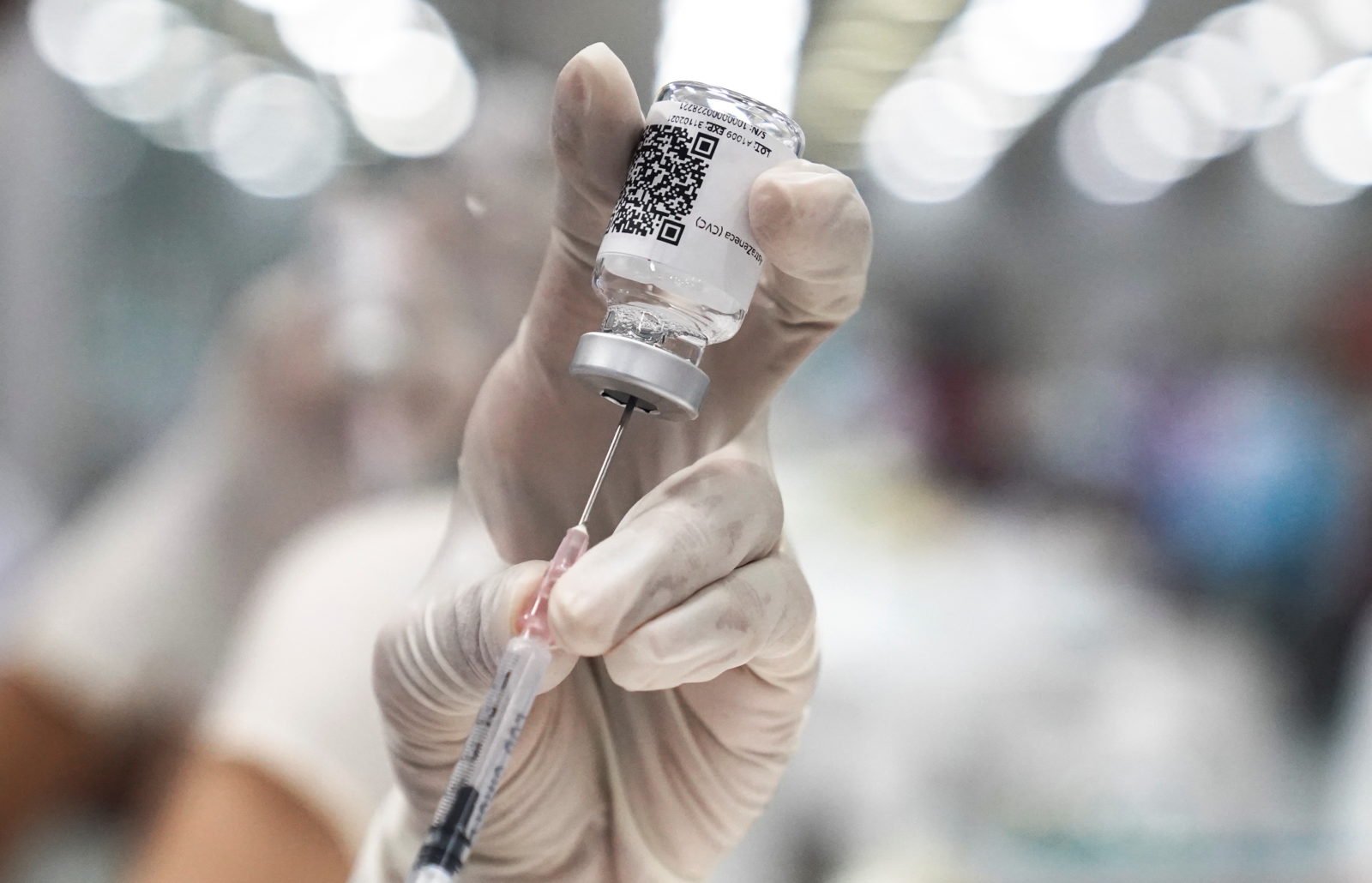
Representative: A health worker prepares a dose of the AstraZeneca COVID-19 vaccine at a clinic in Bangkok, June 21, 2021. Photo: Reuters/Athit Perawongmetha
A preprint paper of a new study, posted on July 16, 2021, by a team of scientists led by Dr Pramod Kumar Garg of the Translational Health Science and Technology Institute, Faridabad, has reported that two doses of the Covishield COVID-19 vaccine was 63% effective against the delta variant of the novel coronavirus.
What was this study and how reliable are the results? Let’s find out.
India’s COVID-19 vaccination drive depends heavily on Covishield, the vaccine manufactured by Serum Institute of India, Pune, under license from the British-Swedish pharmaceutical firm AstraZeneca. Both Covishield and the AstraZeneca vaccine are the same recombinant vaccine, christened ChAdOx-1.
Thus far, we have taken at face value the claim that the findings of the major foreign clinical trials (such as those published in December 2020 and February 2021) using the ChAdOx-1 vaccine apply equally to Covishield. Technically, there is no reason why they should not – but as the virus evolves, it is prudent to keep making sure the two vaccines are equivalent, as well as measure their efficacy against the new variants.
The July 16 preprint paper, of a new study from India, is a welcome development on this front. It is a complex paper because it describes two separate pieces of research. This article focuses solely on the case-control study that reports the vaccine’s effectiveness in the field. The same paper also describes the action of antibodies generated by Covishield against the delta variant. But this article will not comment on this part of the study, to keep things simple.
How vaccines are tested
The phase 3 trials of vaccines are randomised controlled trials (RCTs) – the gold standard of clinical assessment techniques, and based on data from such a trial, they calculate a vaccine’s efficacy. The study by Dr Garg was not an RCT, however, but a ‘test-negative case control study’.
In an RCT, researchers start with participants who have not had COVID-19, divide them into two groups at random. One group receives the vaccine, the other receives a placebo. The researchers then follow up with both groups, recording the number of COVID-19 infections that occur in each group.
Because the participants have been assigned to the placebo and vaccine groups at random, differences in the virus’s ‘attack rate’ between the two groups can be attributed to the vaccine. If the attack rate in the vaccine group is, say, 20% of the attack rate in the placebo group, we can say that the vaccine prevented 80% of the infections that would have occurred without the vaccine. The 20% in this example is the ratio of the risk of getting COVID-19 between the two groups – and the 80% is the vaccine’s efficacy.
RCTs of this kind are expensive, take time and, once vaccines have been approved – even if only for emergency use –, are difficult to repeat. This is where case-control studies can be useful.
Here, researchers start with a group of people who are grouped as ‘cases’ and ‘non-cases’, or ‘controls’. They then quiz the subjects in each group to determine whether they have received the vaccine in the past, to get a sense of the vaccine’s efficacy.
For example, let’s say we had 1,000 people who had COVID-19, of which 50 had received the vaccine, leaving 950 unvaccinated. So the odds of a person in the ‘case’ group having had the vaccine is 50 in 950, or 1 in 19. The odds of people being vaccinated in the ‘cases’ and ‘controls’ groups are compared to yield the odds ratio (OR).
Dr Garg and his team studied people who had been tested for COVID-19 with the RT-PCR test in one of two centres in Faridabad, Haryana, between April 1 and May 31, 2021. In this period, a second COVID-19 wave was surging in India and the pace of vaccinations was only just picking up.
Dr Garg’s team selected 3,687 people who tested positive on RT-PCR tests and 5,163 people who tested negative. After leaving out those who didn’t respond and those who had to be excluded for other reasons, both groups shrank to 2,766 positives and 2,377 negatives.
Next, the team went back in time[footnote]Figuratively – not in a time machine[/footnote] and asked the participants whether they had received the vaccine, and if so, how many doses they had received.
This is the real attraction of the case-control method: since we start with the outcome – ‘case’ or ‘non-case’ – and look back in time, it offers a relatively quicker way to assess the vaccine’s effects on a population. Such assessments could proceed even faster if all this information was readily available in a clinical database.
The data from the study is summarised in the table below. The figures come from table 2 of the paper.
‘Cases’ (RT-PCR +ve) | ‘Controls’ (RT-PCR -ve) | |
Vaccinated – two doses | 85 | 168 |
Unvaccinated | 2294 | 1813 |
Odds of vaccination | 85/2294 = 0.0370 | 168/1813 = 0.0926 |
Odds ratio | 0.0370/0.0926 = 0.3995 |
Note that 0.3995 is this author’s estimate of the odds ratio. In the paper, the researchers have presented an adjusted odds-ratio – accounting for age, sex and COVID-19 exposure risk differences, although they don’t provide details of how they arrived at their final figure, of 0.37. But it is not far from 0.3995.
Interpreting the numbers
It’s hard to intuitively make sense of the odds ratio. Mathematically speaking, it is not the same as the relative risk or the risk-ratio calculated based on a forward-looking clinical trial. Instead, and broadly, it measures the strength of a causal link between exposure to the vaccine’s protective effects and the outcome, which in this case is ‘getting COVID-19’.
Where an outcome is infrequent or rare, then the OR becomes a sensible approximation of the relative risk. The OR here is lower than 1, which means the vaccine does have a protective effect.
A vaccine’s efficacy is 1 minus the relative risk, and its effectiveness is 1 minus the OR. On this basis, Dr Garg and his team arrived at an estimated effectiveness of 0.63 – or 63% – for two doses of Covishield.
Now, first: the researchers’ claim that their study addresses Covishield’s effects against infections of the delta variant based on sequencing only 150 samples from the cohort of 2,766 participants (‘cases’ group). Of these, 121 (80.7%; 95% CI 73-87) samples contained the delta variant or variants of its lineage. Another 17 could not be assigned to any lineage. Notwithstanding the paucity of genome-sequencing activities in India, it is not unreasonable to conclude that most of the 2,766 people in this study had COVID-19 caused probably by the delta variant.
Second: the researchers presented data vis-à-vis mild and severe COVID-19. Of the 84 participants with severe disease, only one had been vaccinated. However, they contrast these odds, of 1 in 83, not with the ‘controls’ but with the mild cases (with odds of being vaccinated of 1 in 26), to arrive at an OR of 0.19.
This translates to an estimated effectiveness of 81.5%. But because of the small number of people with severe COVID-19, this estimate comes with a very wide 95% confidence interval[footnote]The range across which a given value can vary, with ‘95%’ being a qualitative threshold[/footnote] – of 9.9% to 99%, making it wholly unreliable! In any case, the OR for severe disease should have been calculated against the odds in the control group. Doing so yields an OR of 0.13, with a similarly giant confidence interval of 0.018 to 0.94.
Third: in any case-control study, the biggest source of bias is in identifying the controls. Here, the authors used a standard WHO Excel tool to calculate the ideal sample size, based on standard assumptions, of 2,237 ‘cases’ and an equal number of ‘controls’. In the final analysis, the authors included 2,766 ‘cases’ and 2,377 ‘controls’ – both comparable to the estimated ideals.
However, this is not the number they started with. Remember that they contacted a total of 8,850 people.
‘Cases’ (RT-PCR +ve) | ‘Controls’ (RT-PCR -ve) | |
Contacted | 3,687 | 5,163 |
Responded | 3,086 | 4,036 |
Exclusions | 320 | 1,659 |
Included in final analysis | 2,766 | 2,377 |
The paper itself presents this information in a somewhat confusing manner.
It is of some concern that the non-responders and exclusions amounted to 25% among the ‘cases’ originally contacted and twice that number – 54% – among the ‘controls’. The researchers write in their paper that the exclusions happened for “reasons mentioned in the supplementary material”, but this author couldn’t locate such material on the preprint repository’s website.
Whenever a large fraction of participants enroll but then drop out before the final analysis, there is room for reasonable doubt about a study’s integrity and conclusions. We have to assume that the participants who dropped out or were excluded are similar to those who were included in the final analysis, so that the conclusions remain valid. But in this case, the loss to follow-up was almost twice as large in the ‘controls’ group as in the ‘cases’ group. This means we have to make a leap of faith to assume that this lopsided loss rate didn’t affect the direction and size of the estimated OR.
To compare, consider the May 2021 study by Public Health England. Here, vaccination data was available for 90% of the nearly 175,000 people who underwent RT-PCR tests. The researchers also had data of 44,950 people who tested positive (‘cases’) and 112,340 who tested negative (‘controls’). This study was conducted entirely by linking participants’ records across databases of RT-PCR tests and vaccinations. India lacks such routine and comprehensive health-related databases. However, researchers have performed good case-control studies for our benefit.
To repeat, case-control studies are useful when a disease or an outcome of interest is rare. They offer useful insights into potential causal links between important factors like vaccination and disease risk. But the key in these studies is to properly select the ‘controls’ and ensure the data is complete. When it loses half the ‘controls’ it started out with, the conclusions’ reliability becomes suspect.
Dr Jammi Nagaraj Rao is a public health physician, independent researcher and epidemiologist in the UK.